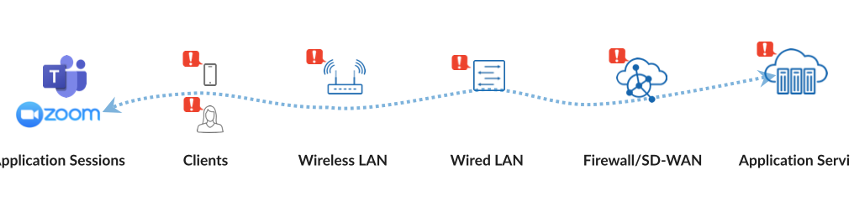
What does AIOps mean for the networking world?
I’ve previously written about the buzz around AIOps and the different points of opportunity through which AI can improve IT operations—whether it’s in detecting fraud, eliminating downtime or planning for capacity. Today, let’s take it one step further and look at how AI can be applied to the network.
The network is the foundation for all applications. With the increase in distributed applications and their hybrid nature, the network has become even more important. You may not realize this, but every application you use goes through different segments of the network. For example, when you click to join a Zoom call, it’s like magic—a few seconds and you’re in the meeting room. However, what happens on the back end isn’t as simple. Once you begin to look at the many different components that are required for a reliable call with no degradation of video or audio quality, it’s quite impressive. A Zoom call, or any application session for that matter, first hits the Wi-Fi access point (assuming you moved to the 21st century and cut the cords already), and travels through wired LANs and SD-WANs (firewalls, gateways, switches, routers, etc.) before it reaches the application services. In that journey, your request (or packet) will compete against other users watching movies (or cat videos), playing games, chatting, shopping, or just wasting time browsing the internet. Add a multi-vendor network footprint to the mix, and it only adds to the complexity.
How does AIOps help solve this complexity?
At the end of the day, AIOps is about providing high Quality of Experience (QoE) to the end-users. Providing a network connection alone is insufficient in ensuring a good end-user experience. Network operators must think about providing reliable, predictable and optimized network flows. A major theme that most companies miss is that IT operational success is not just about avoiding downtime and device/network failures—it goes beyond that. Instead, it is about being proactive and predictive to stay one step ahead of the end user. This requires optimized and cost-efficient network operations. AIOps is well suited to this task because it leverages historical and current data to isolate and predict issues causing poor user experience.
Can AIOps really do that?
As an example, when Juniper decided to use Marvis (Juniper Network’s virtual network assistant powered by AIOps) the AIOps solution, it was able to handle 75% of the service desk tickets without human intervention. Imagine the power of that! Your support teams need to take care of only 25% of the tickets while AI can solve 75% of the tickets, faster than your support teams can.
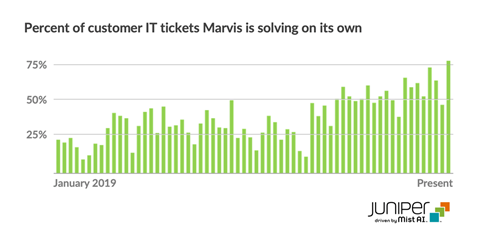
As Marvis continuously ingests more information and data, it learns how to act to fix repeat issues before users know they exist. It can also learn about the new devices added to the network. You don’t have to linearly grow support teams with the growing volume of devices and tickets, when you use AI to handle majority of the support tickets.
This is a testament to the power of AIOps—it can enable smarter remediation and resolution. Delegating more and more tickets to AI will not only help reduce pressure on support team resources, but also fundamentally shift operations from being focused on reactive troubleshooting to proactive remediation.
What are the core AIOps use cases in networking?
- Dynamic baselining & thresholding
AI is best at sifting through troves of data to figure out patterns. In networking, AI figures out various thresholds based on hourly, daily, seasonal and trends-based abnormalities. In the past, this was done using rules-based thresholding which does not take promotional-based, weather-based, or other dynamic user behavior-based traffic increases into consideration. AIOps considers the multivariate-based anomalies and figures out the real deviations for increased demands in services. By doing so, AIOps systems can dynamically adjust the bandwidth rather than restricting the usage of services due to high demand.
- Anomaly detection
Networks today are diverse, comprised of old and new devices. To coexist, operators must pay careful attention to issues around technical debt. For example, some network devices may have been brought in up to 20+ years ago and will not be able to fully support the “software-defined” nature of today’s networks. So instead of the devices themselves reporting and/or helping to find the cause, network engineers will need to rely on looking at the data and detecting anomalies in time series data. AIOps technology can easily search and isolate problematic devices at speed near real time.
- Event correlation & noise reduction
As any network operator can tell you, the volume of data coming out of networks today is huge. For example, a down Wi-Fi access point can create thousands of alerts that overwhelm support teams. Often, it is difficult to find out if those events are even related before the support teams start chasing the issues. AIOps can consolidate data from multiple sources, correlate the events, reduce noise and create only the necessary service tickets. It can also help group service tickets so the same support personnel can look at the related tickets together. This helps reduce “alert fatigue” and allows support to concentrate time and resources only on the necessary events.
- Root cause analysis
This is one of the primary use cases for AIOps. Going through thousands of tickets, alerts and notifications created by a single cause, and exploring the petabytes/terabytes of data to figure out what might have caused is almost inhumane, but this is where AI shines. Based on the time stamping and sequencing of events, AI can figure out in a matter of seconds what happened first and what caused it. Not only can AIOps build an effective causality analysis, but it can also recommend what can be done to fix it. And if equipped properly, AI can automatically handle the remediation. This results in a reduction in Mean Time to Incident Identification, which can reduce the very important metric, Mean Time To Resolution (MTTR).
- Observing Quality of Experience (QoE)
The reality is that today, most network bandwidth apportionment happens manually. With AIOps, systems should be able to measure the quality of performance from vantage points, whether real user based Real User experience Monitoring (RUM), or simulated (Synthetic). Based on what AIOps sees, it can adjust the bandwidth accordingly. AIOps systems can also predict the specific application/systems usage at certain times of day to allocate more bandwidth to those applications and reduce bandwidth to other applications without necessarily increasing the capacity of the network. This will result in highly-optimized network usage at the same (or reduced) cost.
- Self-Driving Network™
Once a network is truly set up to figure out QoE and predict performance degradation, it will be easy to become self-fulfilling. If AIOps is set up to identify real incidents that cause anomalies, identify root causes quickly, and make remediation recommendations, then it should be possible to resolve those problems before they’re even noticed by end-users or even Ops teams. A properly set up “software-defined” network should be able to self-correct for maximum uptime, fix degraded performance, and optimize bandwidth.
- Contextualization of incidents
Once an incident happens, the first step the Ops team is to figure out the context (what, when, and why?). AIOps is able to add and deliver this context to the tickets instead of just notifying when a specific network is down. It can also capture data before and after a network incident and add that context to the service ticket to help visualize what happened. Based on the knowledge base and prior experience, it should also offer remediation possibilities if it cannot be self-corrected. This will allow for easy sharing of information across support personnel rather than the first-level support level having to manually enter subjective information. AIOps systems can also be set up to forward the ticket to the real SME instead of sending everything to L1.
Now that you know what AIOps can do for your network, you should also know how to spot fakes in this new world of AI. In the follow on to this blog, I will cover what an actual AIOps solution for networking looks like and how customers are vetting these solutions with efficacy data and Proof of Concepts (PoC) so they don’t get stuck holding the bag.
If you want to dig into more details check out this blog written by Juniper Network’s head of data science, Jisheng Wang: AIOps: It’s Time the Algorithm Worked For You.
This post is sponsored by Juniper Networks.