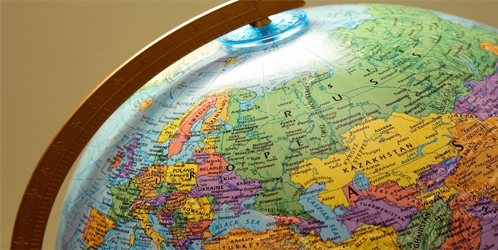
Why we need more control over where our sensitive data is stored, analyzed and processed
Venture Beat
Image Credit: Shutterstock
Maintain your employer brand in a pandemic
Read the VentureBeat Jobs guide to employer brandingDownload eBook
Andy Thurai is chief architect and CTO of Intel App security and Big Data (@AndyThurai). David Houlding is privacy strategist at Intel (@DavidHoulding).
These days, everyone seems to want our personal data. Companies use it for big data analytics, the government’s checking it for suspicious behavior, advertisers want to profile us, and web technologies want to track where we go online and what we do.
As a result, individuals and companies worldwide are seeing higher risks of invasions of privacy, identity-based attacks, and security vulnerabilities than ever before.
This has prompted a number of countries to review and discuss revoking or revising the data protection laws that govern trans-border data flow, such as the EU Safe Harbor, the Singaporean government’s privacy laws, and Canadian privacy laws. The business impact to the world cloud computing industry is projected to be as high as $180 billion.
With data privacy laws varying by country and region, location transparency and the data-subject’s ability to choose the location processing is going to be key to building trust. This includes the location of data centers and cluster nodes that store and process the sensitive personal information of users. While most big data providers are able to provide security for the storage and transmission of sensitive data, most implementations that we see don’t provide location transparency or location-contingent data processing.
Brands that establish and build trust with users will be rewarded with market share, while those that repeatedly abuse user trust with privacy faux pas will see eroding user trust and market share.
Disaster waiting to happen
As major new privacy-sensitive technologies emerge — such as wearables (think Google Glass and Fitbit) and the Internet of Things — data privacy and the availability of identity-protected data processing are likely to become even more important. Providing transparency and protection to users’ data, regardless of how, or where, it is stored or processed, is the key to establishing and building user trust. This can only happen if providers are willing to be transparent about where they process data.
Providing corporations and their target consumers with visibility into where and how their information is processed can establish and build trust. Imagine the power of users being able to choose where their data is processed, or stored, as opposed to being at the mercy of the big corporations and data consolidators. User visibility into favorable location of processing could also be a positive service differentiator in a highly competitive market. One could envision rule-driven big data/analytics where the location of processing of sensitive personal information is also a function of processing locations, country rules, user locations and choices/consent options, and policies.
How can it be solved?
Here is an ideal way to do it: Given the multi-node processing capabilities of big data, you should be able to choose where you will be processing certain data from certain user bases. Users should be able to indicate their preferred security level for processing their data. Data subjects will have visibility into where it is being processed and governance objects will have visibility on how to route data based on user profile and policies, as it knows the secure zone levels and the associated policies.
Given today’s technology, it is possible to build more secure clouds (including using technologies that verify a known clean state that is free of malware and viruses) and have some of the big data nodes process the data more securely from within such highly secure clouds.
Conceptually, GRC (Governance, Risk, and Compliance) collects the location of data subjects and processing resources. GRC, armed with location information, policy rules, and data-subject-choices, can route information based on the locations of both the data subject and processing resources and the level of security of the processing resources. Data can be scrubbed and protected before entering a Hadoop cluster or for data leaks at the API level. This will help with either redacting or masking the sensitive data leaving APIs or entering Hadoop clusters.
People could specify their preferred location and level of security of processing. For example, a person in Germany participating in an online service that involves big data/analytics, perhaps for targeted advertising, prefers for their data to be processed in Germany with a higher level of security. In this case the data center, or Hadoop cluster nodes, used for processing of their data is routed to be processed on a high security compute environment in Germany. Another example could include controversial services such as online gambling, where data subjects prefer any processing of their sensitive personal information to occur in places where those services are legal. The level of processing security in these cases would take into consideration the value of the particular data and associated risk along with the preferred choice of consumers.
We propose data classification levels tagging scheme to enable routing, such as “highly secure processing, geo-tag restricted, medium or none.” For example, data tagged “none” will be executed in the next available cluster, regardless of the location, in the fastest, cheapest possible way. This could also enable service providers to charge based on the classification level as well. For example, if you guarantee enterprise-grade secure processing, then you can charge a high premium to go with that. Geo-restricted labeling would make sure the processing happens within a specific country or (such as EU zone) location. History of data movement and processing can be audited, tracked, and tuned to fit specific needs.
We can also use this approach to enable the service provider to enforce the cleansing operation based on the location. For example, if it is processed somewhere that is not considered a higher security location, destroy the data objects and clean up any residues after the operation.
This is an enhancement we are proposing to our big data group. Subsequently, we hope to influence all versions of big data.
This article was originally published in Forbes on Feb 2, 2021 – https://venturebeat.com/2013/10/02/location-specific-privacy/