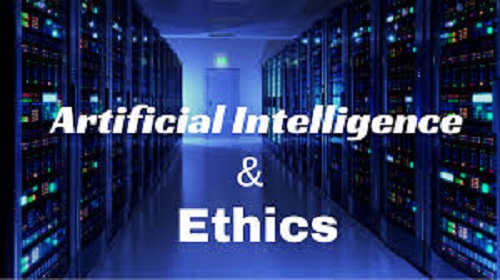
It is Our Responsibility to Make Sure Our AI is Ethical and Moral
A group of teachers successfully sued the Houston Independent School District (HISD) in 2017 claiming their Fourteenth Amendment rights were violated when the school district used an opaque artificial intelligence (AI) algorithm to evaluate and terminate 221 teachers. The judge overturned the use of AI algorithms suggesting, “When a public agency adopts a policy of making high stakes employment decisions based on secret algorithms (aka, AI and Neural Networks) incompatible with a minimum due process, the proper remedy is to overturn the policy.”
The fields of computer modeled risk assessment and algorithmic decision making have been around for a while, but AI takes it to the next level – as demonstrated by Cambridge Analytica’s recent infamous work. AI is having an even bigger impact in our lives than that we find in movies like Terminator and I, Robot. While those movies suggest that the robots might end human freedom and control us – the biased, unfair, or downright unethical decision-making algorithms, automatically created and used by machines, pose a bigger risk to humanity.
In another example, a system called COMPAS — a Machine Learning (ML) based risk assessment algorithm by Northpointe, Inc, — is used across the country by our courts and correctional facilities to predict the likelihood of recidivism (someone who will re-offend) – much like the movieMinority Report. A ProPublica analysis of these recidivism scores revealed a bias against minorities who ended up being denied parole.
When for-profit organizations try predictive law enforcement based on limited and/or biased sets of data, it can go against our constitutional principles. It creates large ethical problems when decisions like loan approvals are based on these algorithms. A growing number of AI researchers are concerned about the rate of growth of biased AI systems. Major corporations such as IBM, Google, Microsoft are working on research programs on how to mitigate, or eliminate, the AI bias. There are about 180 human biases that have been identified and classified, many of them making their way into AI design.
Strangely enough, companies are willing to trust mathematical models because they assume AI will eliminate human biases; however, these models can also introduce a set of biases on their own if it goes unchecked.
Issue #1: Model based on biased data – Garbage In, Garbage Out.
If an AI model is trained using biased data, it will undoubtedly produce a biased model. AI systems can only be as good as the data we use to train those models. Bad data, knowingly or unknowingly, can contain implicit bias information – such as racial, gender, origin, political, social, or other ideological biases. The only way to eliminate this problem would be to analyze the input data for inequalities, bias, and other negative information. Most organizations spend a lot of time on data prep but mainly concentrate on preparing the data format and quality for consumption, but not on eliminating bias data.
Data needs to be cleansed from known discriminatory practices that can skew the algorithm. Also, the training data needs to be stored, encrypted (for privacy and security), and should have an immutable and auditable mechanism (such as Blockchain) for validation later.
Data should only be included if it is proven, authoritative, authenticated, and from reliable sources. Data from unreliable sources should either be eliminated altogether, or should be given lower confidence scores. Also, by controlling the classification accuracy, discrimination can be greatly reduced at a minimal incremental cost. This data pre-processing optimization should concentrate on controlling discrimination, limiting distortion in datasets, and preserving utility.
Issue #2: Technology Limitation
In the past, we used computers to create mathematical models and solve number problems. There is no grey area when calculating something that is fact-based. The inference and the solution are always the same regardless of the sub-segments. But, when computers are used for inference, making subjective decisions, it can cause problems. For example, a facial recognition technology can be less accurate for people with a certain skin tone, ethnic origin, etc. If the technology is less accurate in identifying the personality and/or profile, how can we account for that? Maybe a secondary algorithm to augment the results, or compensation based on a score booster needs to be done. If a human makes a judgment call (such as a police officer shooting someone), there is a process to validate that judgment call. How can we validate the judgment call a machine makes?
Issue #3: Do more with less
Though our data collection has exploded with the invention of sensors/IoTs, we are still in an infancy stage of data collection. While we have more than enough data for the current state of things, the historical data is still limited for comparison. More and more AI systems are asked to extrapolate the information and make inferences based on subjective decisions. When it comes to AI/ML, more data is always better to identify patterns. But, often there is a lot of pressure to train the AI systems with the limited dataset and continue to update the models as we go along. Can the model be trusted to be 100% accurate based on the limited datasets? No system or human is 100% accurate, but to err is human. Can the machines afford to err? And, if they do, are we divine enough to forgive them?
Issue #4: Teaching human values
This is the most concerning part. IBM researchers are collaborating with MIT to help AI systems understand human values, by converting them into engineering terms.
Stuart Russel pioneered a helpful idea known as the Value Alignment Principle that can help in this area. “Highly autonomous AI systems should be designed so that their goals and behaviors can be assured to align with human values throughout their operation.” It teaches “value alignment” to robots by training them to read stories, learn acceptable sequences of events, and understand successful ways to behave in human societies.
Specifically, the Quixote technique proposes aligning an AI’s goals with human values by placing rewards on socially appropriate behavior. It builds on prior research called the Scheherazade (a system which works on the basis that an AI can gather a correct sequence of actions by crowdsourcing story plots). Scheherazade learns the “correct” plot graph. It then passes that data structure along to Quixote which converts it into a “reward signal” that reinforces certain behaviors and punishes other behaviors during trial-and-error learning. Quixote learns that it will be rewarded whenever it acts like the protagonist in a story instead of randomly or like the antagonist.
Issue #5: Validate before deployment
As the old proverb says, “Caesar’s wife must be above suspicion.” By trusting opaque decision making to systems that even have an iota of suspicion will only erode the trust between humans and machines, especially when machines move from being programmed on what to do to an autonomous self-learning and self-reasoning mode.
This is where AI itself can help us. Researchers are working on a rating system that ranks the fairness of an AI system. Unconscious biases are always a problem, as it is very hard to prove intent. This can lead to unintentional outcomes based on subjective inferences. Until the day AIs can self-govern, there should be a system in place to analyze, audit, validate and be able to prove that the decisions made are fair without any bias.
As Reagan’s popular quote “Trust but verify” suggests, you can trust the algorithm will do the right thing, but make sure the algorithm is validated using other mechanisms before it is deployed. While the human biases can be broken by setting corporate/society values, value training, and having procedures in place, machines need a different approach. Produce auditable, verifiable, transparent results so that the AI system is unbiased, trustworthy, and fair. Build AI systems that continually identify, classify, re-train, mitigate, and self-govern.
Issue #6: Organizational Culture, Training and Ethics
Perhaps the most important issue is to change culture, process and training. Leadership needs to set the ethical tone. While the use cases and regulations can drive the specific architecture, security, etc., the investment and commitment need to come from the executive leadership. They need to set the tone on doing the right things all the time – even in for profit organizations.
As the current political events point out, by working towards a race, gender, color or other fact-based inequality, you will not create greatness: you will end up creating a divisive, sub-standard mentality that in the future ends up hurting the society instead of helping it. Building a fair AI system might help eliminate human-bias and eliminate subjective decisions, but make sure you build a fair AI system that eliminates a machine bias as well.
While AI may not result in the rise of the machines leading to post-apocalyptic scenario, its potential to skew society is equally terrifying. It is our responsibility to make sure there is enough checks and balances to ensure our AI is ethical and moral.
References:
1. Algorithmic Impact Assessments: A Practical Framework for Public Agency Accountability
2. Towards Composable Bias Rating of AI Services – Biplav Srivastava and Francesca Rossi
This article was originally published in AI trends on Jan 15, 2019 –