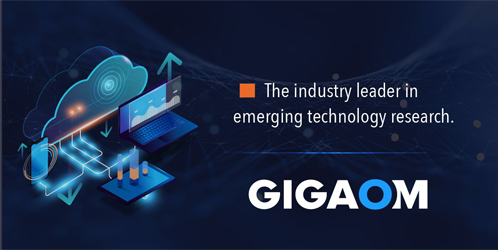
How To Rise Above The ITOps Chaos Using AI
CIOs (Chief Information Officers) are both excited and scared about digital transformation and the pace of innovation. While the ability to drive forward their businesses using IT is exciting, along with the agility and flexibility of newer IT infrastructure models, the fact that business will come to a standstill if information technology (IT) services go down is a scary proposition. Yet, most enterprises are trying to fix problems after they occur instead of preventing them from happening in the first place.
Gartner estimates that the data volumes generated by IT infrastructure are increasing two-to-three-fold every year. This combined with shrinking IT operations budgets is a clear recipe for disaster. Using artificial intelligence (AI) can perhaps solve this problem.
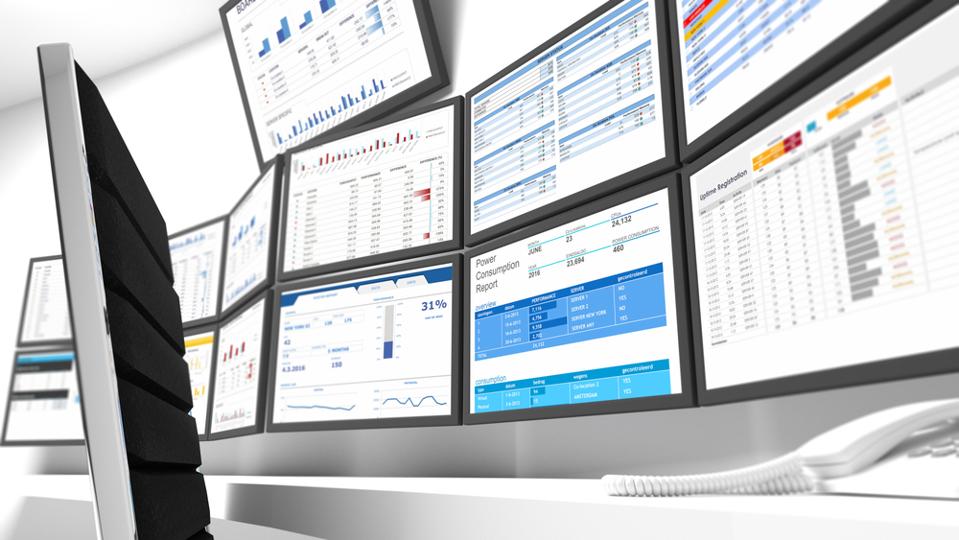
DEEPSENSE.AI
Today’s hybrid IT infrastructure comprises a mix of many stable on-premise and fast moving on-cloud elements: services that use fast-moving components such as micro services, (lambda) functions, and scaling compute power as needed. While this agility helps with bringing innovation to production much faster, this can often create a chaotic environment for IT operations teams. When it comes to IT services, a small component collapsing at a strategic location can cause major havoc. Often times, the failure of even the smallest of components can cause a complete business service outage. For a business service to operate flawlessly, all the components in multiple cloud/data center (DC) locations need to be monitored, managed, alerted, maintained, and acted upon in real time. However, that can lead to data overload.
“It won’t happen to me” syndrome
Many CIOs think such an outlier of disaster will never happen to them. However, a quick look at downdetector.com shows that enterprises big and small have all had major and frequent IT outages. Let’s take a closer look at two major incidents that cost enterprises dearly in the last few years.
In July 2016, when a critical router failed at a Southwest airline data center, it resulted in 2300 canceled flights, almost 10% of their weekly flights, costing the airline nearly $60 million in lost revenue. The outage was fixed in about 12 hours, and the IT Ops crew had the airline systems up and running. However, that was only the IT side of the recovery; it left a mess of thousands of stranded passengers, crews, and planes all in the wrong places. It took weeks for the airline operations to return to normal. All this had been caused by a failed router. The original outage was said to be because of an overheated power supply. Those components were probably monitored, and the alerts were almost certainly buried along with many hundreds of thousands of alerts they had received around that time when the disaster occurred.
In 2015, the NY stock exchange had a major outage that lasted nearly 4 hours. It cost them an estimated $42 million in lost revenue. They also were fined $14 million by the federal regulators because the outage crippled the NYSE trading floor. This was caused by a connectivity issue involving two network gateways not communicating with each other after a software update.
These are just two examples of an alarming rate of IT operations failures in the recent past. Every year, IT downtime costs an estimated $26.5 billion in lost revenue.
While the associated estimated cost is mostly in revenue loss, the damage to the brand reputation can be even higher, especially in highly-competitive industries; customer churn can be high because of these incidents. What is worse, after fixing the current issue, enterprises often fail to implement preventive measures so that such disasters might be avoided in the future.
Prevention is better than cure
In a recent survey of 200 IT decision-makers by Opsview, 81% said they were well prepared and could quickly recover from any major IT disasters. 73% of the decision-makers thought this was the most critical function of IT operations teams, ensuring that their businesses were able to run smoothly.
However, only 18% of them thought they would be ready to continue operating without missing a beat if disaster struck. What is worse, only less than half of them thought avoiding disaster was critical for their company. In other words, almost every major IT company had procedures in place to recover from a major disaster, but only 1/5th of them knew how to avoid a disaster or continue to operate if disaster struck.
When your entire business depends on IT, it is dangerous to worry about recovery instead of prevention. Many enterprises are clearly thinking old school, and they need to start thinking about how to prevent disasters from happening in the first place. “Digital-native” companies rely on IT entirely to run their business (think of Google/Uber/Netflix). By infusing artificial intelligence (AI) into IT operations, every IT-dependent business can avoid these disasters and start thinking in terms of prevention is better than cure.
IT operations are complicated
Almost every major enterprise is now digitized. Every one of them runs hundreds of business-critical applications, which are run on thousands of services, micro-services, and servers. Today’s modern applications are getting more and more complex and often run on multi-location, multi-cloud micro service-based architecture. It is more important than ever to monitor multiple infrastructure domains for a single event.
A disastrous event can create tens of thousands of alerts, signals, events, and triggers across multiple infrastructure domains. Unless you have a mechanism to auto discover, and auto co-relate, across layers of your digital business, the ITOps team will often be clueless and end up chasing thousands of alerts triggered by a single disastrous event.
What can AIOps do for you?
To make faster and better decisions, you need to identify and isolate the problems quickly. When a single event can produce thousands of alerts, the ITOps teams can be lost in searching for a needle in a haystack, or even searching for the right haystack. These problems can’t be solved by adding hundreds of Ops guys to solve this information overload.
Infusing AI into enterprise ITOps (known as AIOps) can help solve the above problem.
Anomaly detection
In a hybrid model, the infrastructure layer is spread across multiple locations. When you layer in various technology stacks that need to be used for each variant (such as cloud vs enterprise data center), the vast amount of data produced can be overwhelming. The normal IT monitoring and alerting systems, which are typically rule/threshold based, can be confused when they encounter a previously unseen problem. Dynamic thresholding can adjust for seasonal, weekly, and daily patterns and alert a human ITOps analyst to look closer into a suspected anomaly in real time.
Because the identification is quick, and the data is co-related, the ITOps teams can work to figure out the root cause in near real-time. While this may not avoid outages, it will reduce the MTTR and have your systems back and running in a matter of minutes.
Noise reduction/Event consolidation
AI can help you reduce a large stream of low-level system events to a smaller number of local incidents. For example, a single logical incident (such as a router failure) can create more than 10,000 network events and many service tickets. AI can auto discover the co-related logs and parse it, detect periodicity of events happening at a certain time, analyze frequent patterns, and do a temporal association detection. By overlaying this on the network topology graph analysis, and with some entropy-based coding, the events can be grouped into minimal logical groups. This can eliminate the volume of event streams up to 95+% of the original volume. This white noise reduction will allow ITOps teams to take a look at a few specific important events instead of looking at an overwhelming number of logs and alerts.
Capacity planning
Using time series forecasting, AI can predict future usage values, such as CPU, memory, server size, network throughput, help desk ticket count, and mean time-to-resolution (MTTR) of incidents. By accurately forecasting the usage ahead of time, even if it were only hours ahead, an enterprise could purchase reserve instances at reduced costs to cope with the demand increase in a cloud-based usage model. In a regular data center situation, the procurement cycle can be sped up and the systems can be ready for demand increase. This can result in large cost savings.
Service ticket analytics
Paradoxically, ITOps teams are struggling with budget restrictions. Managing reduced budget and increased service tickets in a hybrid multi location is an extremely difficult task. You need to accurately forecast how many ITOps analysts are needed at any given time based on an estimation of service tickets adjusted for seasonality and predictable events.
Based on historical data combined with machine learning- such as time series-based modeling, ARIMA, and multivariate analysis – AI can forecast with a high accuracy (up to 95%) on the expected number of service tickets. This will allow for resource allocation suggestions, which can be used to employ a certain number of analysts, support desk help, and customer service personnel for any given day/time.
Conclusion
AIOps machine learning-powered solutions can significantly improve today’s data-heavy IT infrastructure management. AI can help accurately predict the issues before they happen, pinpoint anomalies, locate issues quickly, and reduce MTTR to keep the IT operations running smoothly—all in an automated process.
This article was originally published in Forbes on Feb 8, 2020 – https://www.forbes.com/sites/googlecloud/2021/02/05/6-trends-that-will-shape-the-financial-services-industry-in-2021/?sh=b4803d42b6cb