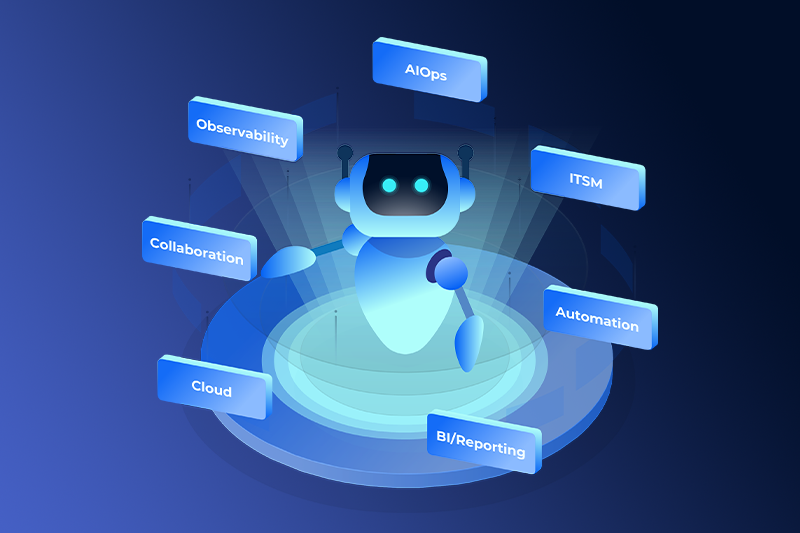
AIOps Has a Data(Ops) Problem
Originally published in APM Digest on May 13, 2021
Modern complex systems are easy to develop and deploy but extremely difficult to observe. Their IT Ops data gets very messy. If you have ever worked with modern Ops teams, you will know this. There are multiple issues with data, from collection to processing to storage to getting proper insights at the right time. I will try to group and simplify them as much as possible and suggest possible solutions to do it right.
Data Volume
Netflix, Uber, and most other digital giants claim to make thousands of “daily production changes.” That is the power of agile development processes. But that is a nightmare for Ops teams. As Ops gurus Gene Kim and Kevin Behr point out in their famous book The Visible Ops Handbook, 80% of unplanned outages are due to ill-planned changes. Changes can be either to application/services or to infrastructure. If 80% of unplanned outages are caused by changes, and if thousands of daily production changes happen regularly, you have no option but to closely monitor any changes to your entire application stack.
But the problem with that is the data volume. Monitoring a decent-sized modern application can produce upwards of terabytes monthly. A complex production application would run on hundreds (or even thousands) of containers, and potentially multiple Kubernetes clusters, and possibly in multi-cloud locations to serve multiple requests per second. If you look at the Netflix architecture picture below, you will get an idea of how complicated distributed monitoring & observability can be.
Data Collection
There are two issues with data collection. The first is proper instrumentation. It sounds easier than it is. The entire observability, monitoring, and AIOps eco-system depend on properly instrumenting your observable sources. If your systems, devices, services, and infrastructure is not properly instrumented, then you will have data blind spots. No matter how much data you collect from certain areas, if you do not have a holistic view of all the telemetry components, you will be getting a partial view of any system. Obviously, the instrumentation depends mostly on developers.
The second issue is integration. As any AIOps vendor will tell you, this probably is the most difficult part to get your AIOps solution going. The more input from varying telemetry sources, the better the insights will be. Any good AIOps solution will be able to integrate easily with the basic golden telemetry – logs, metrics, and traces. In addition, integrating with notification systems (such as OpsGenie, Pagerduty, etc.), and maybe event streams (such as Kafka, etc.) is useful as well. However, I quite often see major enterprises struggling a lot to integrate the AIOps solutions with their existing enterprise systems.
In particular, the need to integrate with several existing enterprise systems such as ITOM, ITSM, CMDBs, and collaboration/service tools can make it even more difficult. An easier way to solve this is to either automate or produce cookie-cutter integrations that can speed up the most time-consuming integration processes.
Data Storage, Transport, and Security
Assuming you process the data at the source, such as in the cloud where it was created, this is not an issue. However, if the data either needs to be transported or stored, it can get quite expensive over time. First, most clouds will charge for data egress, and some clouds charge for data ingress. Assuming your AIOps is not at source, then this cost needs to be taken into consideration as well. Plus, if the data needs to be stored in full fidelity for analysis later, then the costs can be high. For example, Uber built their own cloud-native time-series database M3 to store metrics information, reducing their storage costs by 90%, which is huge when you have large volumes of data.
Data Processing
This is probably the most difficult problem in the data pipeline for AIOps, especially because Ops data comes in multiple formats — some structured, some unstructured, and some semi-structured. In addition, data delivery can also come in different modes and sizes — streaming, batch, bulk queries, notifications/events. The first issue with data preparation involves doing DataOps; integrity checks, data cleansing, transforming, and shaping the data (aggregating, filtering, sorting) needs to be automated to be easily consumed by AIOps systems.
Often, the data inadvertently includes “dirty data.” It could be either missing information, inconsistent information, errors, omissions, or even bias information. Particularly because most AIOps systems use machine learning on datasets, having dirty data can skew the results. It is estimated that an average of 10-20% of the raw data can have deficiencies and need to be made machine-learning ready.
The secondary issue is often that the data lacks application or service context. Operations data is just a piece of a machine or human-generated information. Without context, it will not make any sense and will not give insight as to what is happening in the big picture. To get a deeper understanding, the data should be enriched in real-time with context from other systems and events. It is particularly painful if code needs to be written for every step; for example, a new type of transformation would need a new set of code, etc. Creating, testing, and maintaining that code for every data set is nearly impossible and becomes a nightmare when the number of data connectors grows.
Essentially, building a data pipeline or DataOps is key for any effective AIOps solution. Plus, data also needs to be enriched with contextual information. An isolated incident creating a service ticket will create too much service ticket volume. Instead, a good system should consolidate the tickets and enrich the ticket with additional information, such as corresponding events, correlated incidents, etc., to help support personal resolve issues quickly.
DataOps Can Improve the AIOps Data Pipeline
Both during POCs and productionalization of AIOps, almost all enterprises struggle mightily with streamlining and smoothening the data pipeline for AIOps. I see a major need for enterprise-grade tools in this area. It is a very rough, unorganized, time-consuming, trial and error, and costly process to get this right.
By using self-service automation tools, the data pipeline can be built, experimented with, automated, and put into production by operations teams instead of depending on costly data engineers, data scientists, and database experts. This DevOps revolution to the AIOps pipeline can reduce the dependencies on costly resources and reduce the time to real operational improvement.
Given the complexity of integration, data processing, and data dependency to get the right data to the AIOps to make it more efficient, unless this is fixed, AIOps is not going to produce dramatic results.
DataOps and self-service automation tools are the keys to solving the AIOps data problem. Some AIOps platforms have built-in data pipeline automation abilities to speed up the TTM and to maximize the ROI. Careful consideration of your specific problem needs to be mapped out against the AIOps tool-of-choice instead of picking one based on the popularity of the tool.
If AIOps is not given the right data, you will get garbage results. Fix the AIOps data pipeline first to get meaningful results.
Check out the webinar that I am doing on this topic with CloudFabrix. You can register for the webinar here.
This post is sponsored by CloudFabrix